Chains with Complete Connections
Last update: 21 Apr 2025 21:17First version: 1 August 2016
\[ \newcommand{\indep}{\mathrel{\perp\llap{\perp}}} \]
"Chain with complete connections" is an ungainly name for an important class of stochastic process, closely related to Markov and hidden-Markov models (HMMs).
In an ordinary (discrete-time) Markov process, we have a sequence of random variables \( S_0, S_1, \ldots S_t, \ldots \), where the future is conditionally independent of the past, given the present: \[ S_{t+1}^{\infty} \indep S^{t-1}_{-\infty} | S_t \] Now let's add an additional sequence of random variables, say \( X_t \), where each \( X_{t+1} \) is a random function of \( S_t \) alone, i.e., \[ X_{t+1} \indep \{ X^{t}_{-\infty}, S^{t-1}_{-\infty} \} | S_t \] So far, this is the usual set up for HMMs. What makes chains with complete connections special is that there is a deterministic function, say \( q \), where \[ S_{t+1} = q(S_t, X_{t+1}) \] That is, the next state is determined by the current state and the observation which that state produces. Notice that this means the sequence of pairs \((S_t, X_t) \) is also a Markov process. The \( X_t \) process, however, is not necessarily Markovian, and in fact generally isn't.
The difference between CCCs and HMMs can is perhaps clearer with a graphical model:
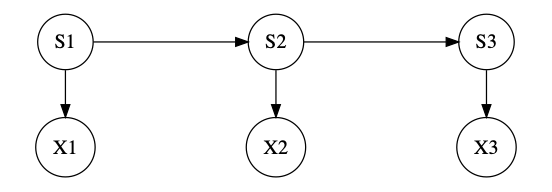
CCC
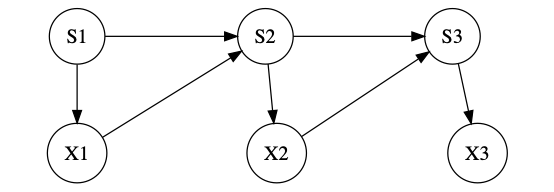
On the other hand, here's an example to show that a CCC isn't necessarily a Markov chain at any order. Take two states, call them 1 and 2. State 1 flips a coin and produces either A or B. If it produces A, it goes back to A; if it produces B, it goes to state 2. State 2 always produces a B, and goes back to state 1. (That is, \( S_t \) can be either 1 or 2, and \( X_t \) can be either A or B.) This, too, can be illustrated by a picture, but it's a state-transition diagram, not a variable-dependence graphical model:
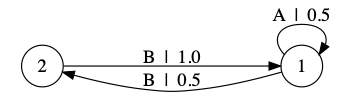
(The first two diagrams used the visual conventions for graphical models, where nodes are variables and arrows indicate direct dependence; this one uses the visual conventions for automata theory / Markov models, where nodes are states and arrows are transitions, labeled with the observations made on each transition. I realize it's bad pedagogy to switch visual conventions from one figure to the next...)
This process will produce blocks of Bs of even length, separated by blocks of As of arbitrary length. But then the conditional distribution of the next observation changes depending on whether it was proceeded by an even or an odd number of Bs, which can't be determined from any fixed, finite history, hence the observable process isn't Markov at any finite order. (The length of history we need for context is however almost-surely finite.) This example is, I was told, originally invented by Benjamin Weiss in the 1970s, and called the "even process", though I haven't verified those references.
I find this class of processes interesting for a couple of reasons. One is that it is very natural for lots of processes, like urn processes, or many models of learning in animals. (Think of the state as being the probability of making different actions, which in turn lead to different rewards, and so to changing the probabilities of those actions.) The other is that just about any stochastic process can be represented in this form, where the hidden states are actually the optimal predictions for the original process. But that subject is involved enough to deserve its own notebook.
--- "Sofic processes" are a special case. (Or, rather, what the symbolic dynamics literature calls right-resolving presentations of sofic processes are.)
--- Cox's 1981 distinction between "parameter-driven" and "observation-driven" models of time series corresponds, in intent at least, to that between ordinary HMMs and chains with complete connections, respectively.
- Recommended:
- D. R. Cox, "Statistical Analysis of Time Series: Some Recent Developments", Scandinavian Journal of Statistics 8 (1981): 93--115 [JSTOR]
- Roberto Fernández and Grégory Maillard, "Chains with Complete Connections: General Theory, Uniqueness, Loss of Memory and Mixing Properties", Journal of Statistical Physics 118 (2005): 555--588, arxiv:math/0305026
- Marius Iosifescu and Serban Grigorescu, Dependence with Complete Connections and Its Applications [Review: Memories Fading to Infinity]
- Bruce Kitchens and S. Tuncel, Finitary Measures for Subshifts of Finite Type and Sofic Systems
- To read, if only I had enough French:
- Octav Onicescu and Gheorghe Mihoc, "Sur les chaînes de variables statistiques", Comptes Rendus de l'Académie des Sciences de Paris 200 (1935): 511--512 [Cited as the paper which introduced chains with complete connections.]
- To read:
- F. Blasques, S. J. Koopman and A. Lucas, "Information-theoretic optimality of observation-driven time series models for continuous responses", Biometrika 102 (2015): 325--343
- Richard A. Davis, Heng Liu, "Theory and Inference for a Class of Observation-driven Models with Application to Time Series of Counts", arxiv:1204.3915
- Randal Douc, Paul Doukhan, Eric Moulines, "Ergodicity of observation-driven time series models and consistency of the maximum likelihood estimator", arxiv:1210.4739
- Randal Douc, Francois Roueff, Tepmony Sim, "Handy sufficient conditions for the convergence of the maximum likelihood estimator in observation-driven models", arxiv:1506.01831
- Roberto Fernandez, Gregory Maillard, "Chains with complete connections and one-dimensional Gibbs measures", arxiv:math/0305025
- Sandro Gallo and Nancy L. Garcia
- "Perfect simulation for stochastic chains of infinite memory: relaxing the continuity assumption", arxiv:1005.5459
- "A general context-tree-based approach to perfect simulation for chains of infinite order", arxiv:1103.2058
- A. Galves, E. Löcherbach and E. Orlandi, "Perfect Simulation of Infinite Range Gibbs Measures and Coupling with Their Finite Range Approximations", Journal of Statistical Physics 138 (2010): 476--495
- P. Ney and Esa Nummelin, "Regeneration for infinite memory chains", Probability Theory and Related Fields 96 (1994): 503--520
- Mohammed Rezaeian, "Hidden Markov Process: A New Representation, Entropy Rate and Estimation Entropy", arxiv:cs.IT/0606114
- To write:
- CRS, "Large Deviation Properties in Exponential Families of Stochastic Automata" [Really, isn't so much "To write" as "To double check and finally kick out something that's been sitting in my agenda folder since around 2006"]